16S profiling analysis pipeline (Windows)
BMP advisory board recommend the use of this pipeline as a standard for 16S rRNA data analysis.
We are now working in order to improve this workflow besides making it easier for end-users.
If you have any questions or suggestions, please contact Victor Pylro: victor.pylro@brmicrobiome.org, Daniel Morais: daniel.morais@brmicrobiome.org or Luiz Roesch: luiz.roesch@brmicrobiome.org
Please, cite our efforts when using this pipeline: Data analysis for 16S microbial profiling from different benchtop sequencing platforms. J Microbiol Methods. 2014. doi: 10.1016/j.mimet.2014.08.018.
​
Please, cite our efforts when using the BTW package: BTW - Bioinformatics Through the Windows: a easy-to-install package to analyze 16S rRNA data on the Windows Subsystem for Linux (WSL). (Submitted).
​
Also, remember to cite all others softwares applied here.
​
VSEARCH
QIIME 1.9
FastX Toolkit
fastq-join
FLASH
ClustalW
RDP Classifier
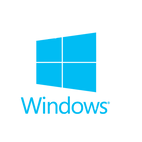
Here, we provide the recommended pipeline for 16S profiling analysis using the Windows Subsystem for Linux (WSL)
What you need: only the BTW package!! Click here
​
Installing the Windows Subsystem for Linux (WSL): Click here
This example assumes reads in FASTQ format.
This page gives a complete pipeline to analyze 16S rRNA gene data. Of course, you should edit as needed for your reads and file locations (represented here as $PWD/).
From Illumina paired-end reads: this pipelines assumes that you have an input folder of paired-up files (by filename, with the default _R1_ and _R2_ containing the forward and reverse reads filenames, respectively):
1 - Take forward and reverse Illumina reads (R1.fastq and R2.fastq files) and join them using the method fastq-join <<<USING QIIME 1.9>>>
multiple_join_paired_ends.py -i input_files -o merged/
​
1.1 - Alternatively, FLASH can be applied to perform the same task (this must be done for each sample, separately)
flash -m 20 -M 250 -x 0.25 -p 33 R1.fastq R2.fastq -o merged/
​
2 - Quality filtering, length truncate, and convert to FASTA each joined sample <<<USING VSEARCH>>>
vsearch --fastx_filter $PWD/fastqjoin.join.fastq --fastq_maxee 1.0 --fastq_trunclen 220 --fastaout samplex.fa
​
Obs: the --fastq_trunclen parameter will depend on the length of you joined reads. You can use FASTQC to taking this decision.
​
3 - Change sequence header to make file compatible with further steps <<<USING BMP PERL SCRIPT>>>. This script will generate your converted FASTA file. Sample´s name should not contain any special characters, symbols or spaces. We strongly recommend keeping samples´s name as simple as possible.
bmp_demultiplexed.pl -i samplex.fa -o samplename -b samplename
4 - Make a single file containing all your samples
​
cat sample1 sample2 sample3 sample4 ... > reads.fa
​
5 - Dereplication <<<USING VSEARCH>>>
vsearch --derep_fulllength $PWD/reads.fa --output derep.fa --sizeout
6 - Abundance sort and discard singletons <<<USING VSEARCH>>>
vsearch --sortbysize $PWD/derep.fa --output sorted.fa --minsize 2
7 - OTU clustering using UPARSE method <<<USING VSEARCH>>>
vsearch --cluster_size $PWD/sorted.fa --consout otus1.fa --id 0.97
​
8 - Fasta Formatter <<<FASTX TOOLKIT SCRIPT>>>
​
fasta_formatter -i otus1.fa -o formated_otus1.fa
​
9 - Renamer <<<BMP SCRIPT>>>
​
bmp-otuName.pl -i formated_otus1.fa -o otus.fa
​
10 - Map reads back to OTU database <<<VSEARCH>>>
​
vsearch --usearch_global $PWD/reads.fa --db otus.fa --strand plus --id 0.97 --uc map.txt
​
11 - Assign taxonomy to OTUS using the RDP Classifier on QIIME (use the file “otus.fa” as input file)
​
assign_taxonomy.py -i $PWD/otus.fa -m rdp -o taxonomy
​
12 - Align sequences on QIIME, using the ClustalW method (use the file “otus.fa” as input file)
​
align_seqs.py -i $PWD/otus.fa -m clustalw -o rep_set_align
​
13 - Filter alignments on QIIME
​
filter_alignment.py -i $PWD/otus_aligned.fasta -o filtered_alignment
​
14 - Make the reference tree on QIIME
​
make_phylogeny.py -i $PWD/otus_aligned_pfiltered.fasta -o rep_set.tre
​
15 - Convert UC to otu-table.txt <<< BMP SCRIPT>>>
​
bmp-map2qiime.py map.uc > otu_table.txt
​
16 - Convert otu_table.txt to otu-table.biom <<< QIIME SCRIPT>>>
​
make_otu_table.py -i otu_table.txt -t otus_tax_assignments.txt -o otu_table.biom
​
17 - Check OTU Table on QIIME.
​
biom summarize-table -i $PWD/otu_table.biom -o results_biom_table
​
18 - Run diversity analyses on QIIME (or any other analysis of your choice). The parameter “-e” is the sequencing depth to use for even sub-sampling and maximum rarefaction depth. You should review the output of the ‘biom summarize-table’ (step 18) command to decide on this value.
​
core_diversity_analyses.py -i $PWD/otu_table.biom -m $PWD/mapping_file.txt -t $PWD/rep_set.tre -e xxxx -o $PWD/core_output
The generated .biom OTU table is also fully compatible with the MicrobiomeAnalyst, a user-friendly web-based platform for microbiome data analyses and visualizations, including taxonomy plots and estimates of α- and β-diversity (http://www.microbiomeanalyst.ca).
​
​​This workflow is under improvement.
​